How AI is Revolutionizing Breast Cancer Detection: A 21% Boost in Accuracy
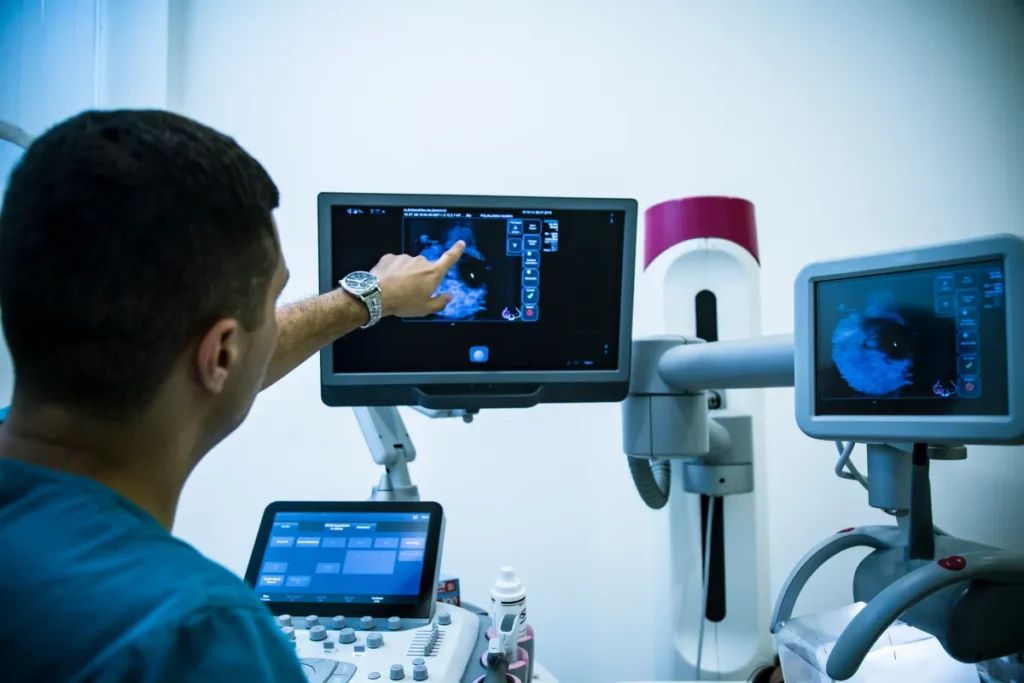
AI in Mammography: Boosting Cancer Detection or Hype? A groundbreaking study presented by DeepHealth, a subsidiary of radiology giant RadNet, suggests that artificial intelligence (AI) could significantly enhance breast cancer detection rates in mammography screenings. According to the data, women who opted for AI-enhanced scans were 21% more likely to have cancer detected than those relying solely on traditional methods. The findings were shared at the Radiological Society of North America’s (RSNA) annual meeting and analyzed a massive dataset of 747,604 women who underwent mammography over a year. For those enrolled in a program offering AI-assisted screenings, cancer detection rates were a striking 43% higher compared to women who opted out. AI as a “Second Set of Eyes” The AI technology, which complies with U.S. Food and Drug Administration (FDA) standards, acted as a supplementary tool for radiologists. Women who paid for this optional enhancement benefited from what researchers called a “second set of eyes.” The AI flagged mammogram anomalies, potentially leading to earlier and more accurate diagnoses. However, not all of the program’s success can be attributed to AI alone. The researchers noted that 22% of the increase in cancer detection was due to selection bias—higher-risk patients were more likely to enroll in the AI-assisted program. Even after accounting for this, the remaining 21% improvement in detection rates was credited directly to AI’s role in increasing recall rates for additional imaging. The Need for More Data While the results are promising, they are far from definitive. The study’s observational nature means the findings are not as robust as those from randomized controlled trials. Researchers are calling for further investigation to “better quantify” AI’s benefits in breast cancer detection and ensure the technology delivers consistent results across diverse patient populations. The Bottom Line The promise of AI in healthcare is undeniable, and this study adds to the growing body of evidence that AI can complement human expertise in critical areas like cancer detection. However, the road to full-scale adoption will require addressing selection bias and proving effectiveness in controlled settings. For now, AI serves as a powerful tool to enhance diagnostic precision—but it’s not yet the revolution some might hope for. As randomized trials roll out, the healthcare community will be watching closely to see if this technology lives up to its potential.
The Economics of AI: Transforming Business, Labor, and Global Markets
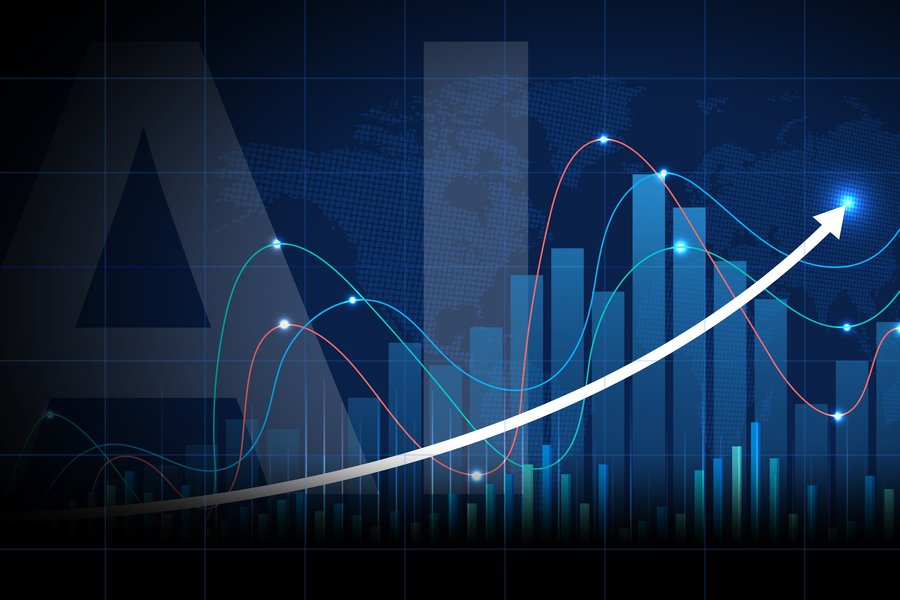
Nobel Laureate Daron Acemoglu on the Real Economic Impact of AI AI is often described as the next great revolution, capturing the attention of investors, technologists, and policymakers. But how much of this vision is grounded in reality, and how much is driven by hype? According to Nobel Prize-winning economist Daron Acemoglu, the answers are unclear. Acemoglu, an MIT economist with extensive experience studying the intersection of technology and society, takes a cautious but insightful look at AI’s economic effects. His focus goes beyond what AI can do to explore what it will do—and who stands to benefit. The Uncertain Economic Impact of AI Despite enormous investments in AI, its long-term effects on the economy remain ambiguous. While some forecasts predict AI could double GDP growth and drive a new era of productivity, Acemoglu offers a more restrained outlook. In his recent paper, The Simple Macroeconomics of AI, he estimates that AI might boost GDP by a modest 1.1% to 1.6% over the next decade, with annual productivity growth increasing by just 0.05%. By comparison, U.S. productivity has historically grown at about 2% per year since 1947. “I don’t think we should belittle 0.5% over 10 years,” Acemoglu says. “But it’s disappointing compared to the promises made by the tech industry.” The Jobs Dilemma: Where Will AI Have the Most Impact? A key uncertainty surrounding AI is its impact on jobs. Studies, including one from OpenAI and the University of Pennsylvania, suggest that AI could affect 20% of U.S. job tasks. Acemoglu agrees that certain white-collar jobs—particularly those involving data processing and pattern recognition—are likely to experience significant changes. “It’s going to affect office jobs that involve tasks like data summarization, visual matching, and pattern recognition,” he notes. However, he emphasizes that many roles, such as journalists, financial analysts, and HR professionals, are unlikely to be entirely transformed. “AI isn’t going to revolutionize everything,” he adds. Automation vs. Augmentation: A Crucial Choice A central theme in Acemoglu’s research is how AI is currently being deployed. Is it being used to enhance worker productivity or simply to replace human labor and cut costs? “Right now, we’re using AI too much for automation and not enough for providing expertise and support to workers,” Acemoglu argues. He highlights customer service as an example, where AI often underperforms compared to humans but remains attractive because it is cheaper. This approach, which Acemoglu refers to as “so-so technology,” fails to deliver significant productivity improvements. Instead, it reduces job quality while saving companies money at workers’ expense. In his book Power and Progress, co-authored with Simon Johnson, Acemoglu explores this dynamic in depth, advocating for innovations that complement workers rather than replace them. This, he argues, is key to achieving widespread economic benefits. Lessons from the Industrial Revolution Acemoglu draws parallels between AI and the Industrial Revolution, noting that technological advances do not automatically lead to societal benefits. In 19th-century England, it took decades of worker struggles to secure fair wages and working conditions before the gains from machinery were broadly shared. “Wages are unlikely to rise when workers cannot push for their share of productivity growth,” he explains. Similarly, the current AI boom could deepen inequality unless deliberate efforts are made to distribute its benefits. The Case for Slower Innovation In a recent paper with MIT colleague Todd Lensman, Acemoglu suggests that society might benefit from a slower pace of AI adoption. Rapid innovation, while often celebrated, can exacerbate harms such as misinformation, job displacement, and behavioral manipulation. “The faster you go, and the more hype you generate, the harder it becomes to correct course,” he warns. Acemoglu advocates for a more deliberate approach, emphasizing the need for thoughtful regulation and realistic expectations. Overcoming the AI Hype Acemoglu believes that much of the current enthusiasm around AI is fueled by hype rather than tangible results. Venture capitalists and tech leaders are investing heavily in AI with visions of sweeping disruption and artificial general intelligence. However, many of these investments prioritize automation over augmentation—a strategy Acemoglu sees as shortsighted. “The macroeconomic benefits of AI will be greater if we deliberately focus on using the technology to complement workers,” he argues. The Road Ahead The future economic impact of AI depends on the choices made today. Will we design technologies that empower workers, or will we prioritize cost-cutting automation? Will we adopt AI responsibly, or rush forward without addressing its challenges? For Acemoglu, the answers lie not only in technological development but also in the policies, institutions, and priorities that guide its use. As he puts it, “AI has potential—but only if we use it wisely.”
Cleerly Secures $106M in Funding to Transform Heart Health with AI
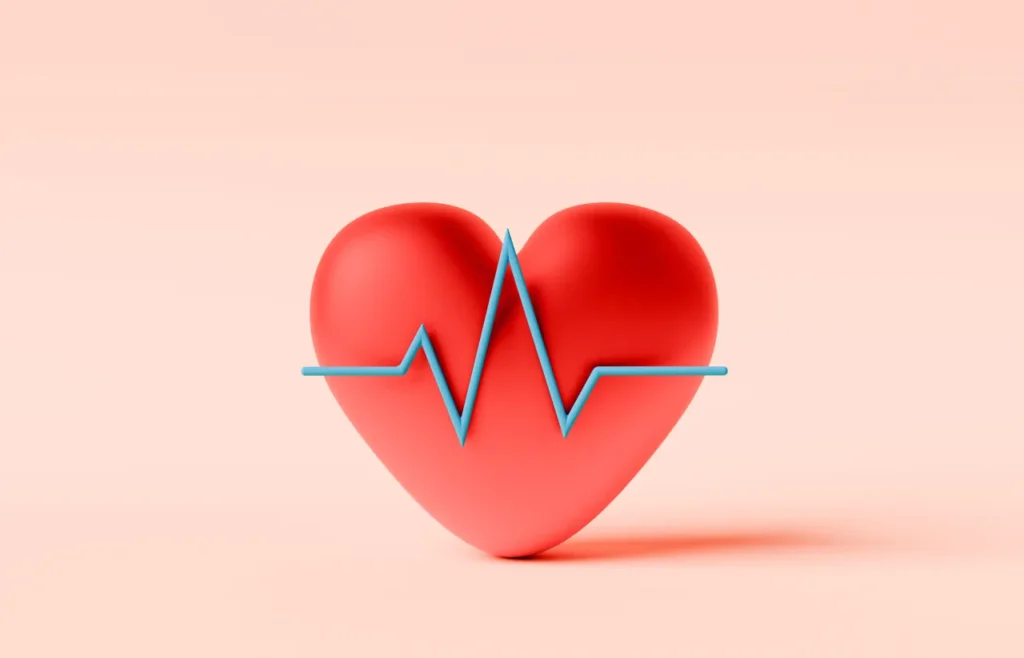
Cleerly’s Vision: AI-Powered Heart Screening to Prevent the Leading Cause of Death Heart disease is the number one cause of death in the U.S., yet many people who suffer heart attacks are unaware they have the condition. Cleerly, a cutting-edge cardiovascular imaging startup, is on a mission to change this. Using AI-powered technology, Cleerly analyzes CT heart scans to detect early-stage coronary artery disease—similar to how mammograms and colonoscopies catch cancer in its early stages. “The majority of people who will die of heart disease and heart attacks will never have any symptoms,” says Dr. James Min, a cardiologist and Cleerly’s founder. “At some point, we need to start screening the world for heart disease.” Founded in 2017, Cleerly emerged from a clinical program Dr. Min began in 2003 at New York-Presbyterian Hospital/Weill Cornell Medicine. Today, the company is conducting a multi-year clinical trial to prove that its AI tools can diagnose heart disease in symptom-free individuals more accurately than traditional methods, such as cholesterol tests or blood pressure monitoring. If successful, Cleerly’s innovative approach could transform heart health diagnostics, creating a new standard in prevention and saving millions of lives. Major Investors Back Cleerly’s Mission Cleerly recently raised $106 million in a Series C extension round, led by Insight Partners, with additional investment from Battery Ventures. This funding builds on the company’s $223 million Series C round in 2021, led by T. Rowe Price and Fidelity. While extension rounds sometimes signal slow growth, Scott Barclay, managing director at Insight Partners, disagrees. “Is growing fast,” he says. This funding helps support ongoing multi-site clinical trials while benefiting from Insight’s expertise in scaling enterprise software. Dr. Min notes that Cleerly wasn’t in urgent need of additional capital but welcomed Insight’s strategic value in scaling the business. How AI Is Changing Heart Disease Diagnosis Cleerly’s technology focuses on identifying plaque buildup in coronary arteries, a key cause of heart attacks. Unlike traditional tests like stress tests or coronary angiograms—both invasive and time-consuming—Cleerly’s AI software analyzes CT scans more efficiently and non-invasively. Health insurers and Medicare have already recognized its benefits. In October, Medicare approved coverage for Cleerly’s plaque analysis test, signaling broad acceptance of this new diagnostic approach. Over the past four years, Cleerly’s commercial availability has fueled growth, with the company reporting over 100% annual growth. With Medicare coverage, Cleerly now has access to roughly 15 million U.S. patients presenting with heart problems annually. Competing for a Healthier Future Cleerly isn’t alone in the AI-driven heart health space. Companies like HeartFlow and Elucid are also developing similar tools. However, the market is vast—millions of individuals could benefit from preventive screening, ensuring room for multiple players. Cleerly’s ultimate goal is to make heart disease screening as routine as cancer screenings. This shift could fundamentally change how the medical community approaches the prevention and early detection of cardiovascular conditions. What’s Next for Cleverly? Although Cleerly’s technology is already FDA-approved for diagnosing symptomatic patients, the company is awaiting clearance for general population screening. If approved, this could open a vast market for its AI tools. Looking ahead, Cleerly aims to prove that its tools not only save lives but also reduce healthcare costs by preventing heart attacks. With growing support from investors, healthcare providers, and payers, is poised to redefine heart health diagnostics. With AI leading the charge, Cleerly is setting a new standard for proactive, accurate, and patient-focused care in the fight against heart disease. Or proactive, precise, and patient-centric heart care.
Photonic Processors: The Future of Ultrafast, Energy-Efficient AI
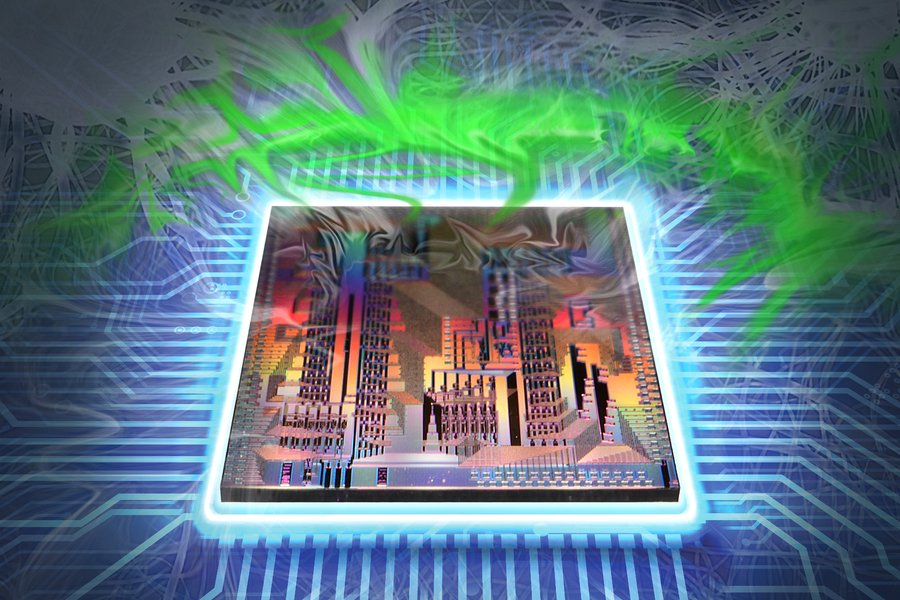
A Breakthrough in Photonic Processors Could Revolutionize AI Artificial intelligence (AI) drives modern innovation, from autonomous vehicles to advanced scientific research. However, as deep neural networks (DNNs) grow more complex, traditional hardware struggles to keep up. Current processors, which rely on electrical currents, face limits in speed and energy efficiency. This is where photonic processors come in. These cutting-edge chips use light for computation. While they’ve shown potential, challenges in handling certain tasks have prevented them from replacing traditional systems. Now, researchers at MIT and their collaborators may have found the solution. A Major Step Forward The team has developed a photonic chip capable of performing all key DNN tasks. This includes nonlinear operations, which were previously difficult to handle with light. Their breakthrough involves nonlinear optical function units (NOFUs). These units combine light and electricity on a single chip. Unlike older photonic systems, this chip doesn’t rely on external processors. It performs all computations internally, making it extremely fast. In fact, it completes key tasks in under half a nanosecond. Even more impressive, it achieves over 92% accuracy, comparable to traditional processors. Why This Technology Matters This new photonic chip offers several game-changing benefits: Beyond AI: Broader Applications The chip’s potential extends beyond AI and machine learning. Its speed and energy efficiency make it ideal for industries such as: Because it can train neural networks on the chip, it could also transform fields that require quick adaptation, like robotics and signal processing. What’s Next? Despite its promise, challenges remain. Researchers need to scale the chip for real-world use in devices like cameras and telecom systems. They also aim to develop algorithms that fully utilize the advantages of photonic processing. “This work shows how computing can be completely reimagined using new approaches that combine light and electricity,” says Dirk Englund, senior author and lead researcher at MIT. If scaled successfully, this photonic processor could redefine AI hardware. It offers the potential for faster, more efficient, and greener systems to meet the growing demands of modern technology. The future of AI computing looks brighter than ever—powered by the light-driven breakthroughs of photonic processors. 4o possibilities are as bright as the photons driving this cutting-edge technology.
How AI is Rapidly Replacing the Tech Support Desk
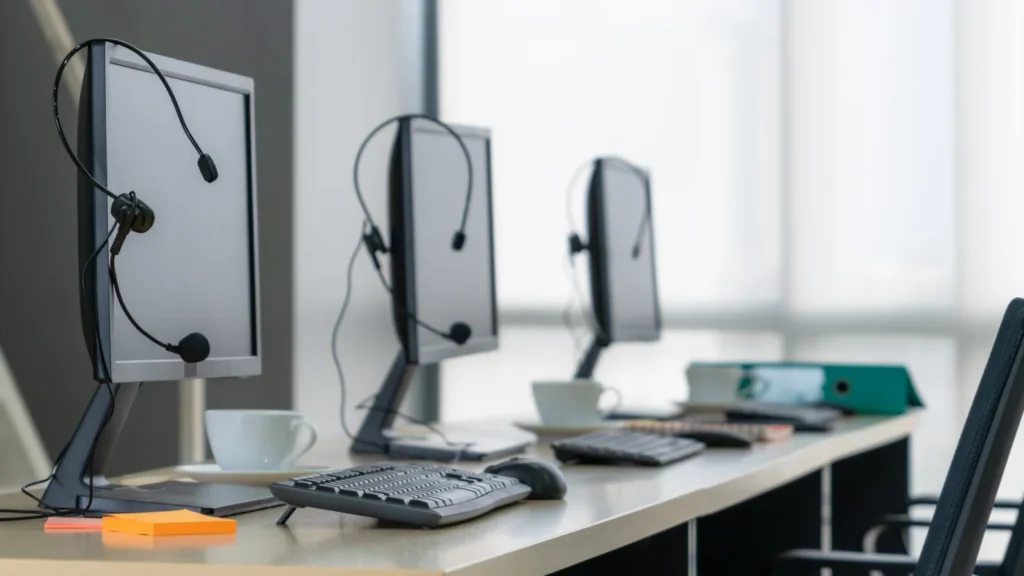
AI Is Reshaping the Tech Support Desk—But Human Expertise Still Matters The Tech Support desk has long been a lifeline for employees navigating the inevitable tech hurdles of the modern workplace. From forgotten passwords to connection issues, these teams have played an integral role in keeping the wheels of productivity turning. However, as artificial intelligence becomes more capable, the traditional IT desk is undergoing a seismic shift, with major companies already replacing a significant portion of their IT staff with AI systems. AI Moves Into the Tech Support Arena Customer-facing chatbots are already familiar, helping with everything from online shopping at Walmart to wearable AI tools used by sales reps at Tractor Supply. Now, internal IT support desks—the ones employees turn to when their laptops crash or their software fails—are the next frontier. Generative AI is poised to dominate this space. By 2027, AI systems are expected to create more IT support and knowledge-base articles than humans, according to Gartner senior director analyst Chris Matchett. This shift fits into a broader strategy of “support case deflection”—automating routine tasks like password resets and making knowledge bases more intuitive. “AI can help streamline these processes,” Matchett said, noting that many companies are already building AI-powered request portals and automated troubleshooting systems. Big Tech’s Experiment: A Case Study At Palo Alto Networks, the transition is already well underway. CEO Nikesh Arora revealed earlier this year that the cybersecurity giant has cut its IT support staff by 50%, replacing them with AI systems capable of resolving most routine tech issues. “We think we can reduce the team by 80% as automation and generative AI continue to improve,” Arora told analysts on an earnings call. The company’s IT support team, which once consisted of 300 employees—or 2% of its workforce—now relies heavily on AI to manage internal tickets. Meerah Rajavel, Palo Alto Networks’ CIO, said the integration of AI has significantly boosted efficiency, particularly in areas like documentation. “AI techniques have accelerated documentation searches by 24 times, thanks to tools like Prisma Cloud Copilot,” Rajavel said. “But the overarching goal remains clear: faster responses, greater accuracy, and a more resilient security posture.” Even with these advancements, Rajavel emphasized that AI still works in tandem with human employees, handling routine tasks so IT teams can focus on higher-level problems. The Human Element: A Sticking Point Despite AI’s impressive capabilities, it hasn’t yet won over all employees. A Gartner survey of over 5,000 digital workers revealed that fewer than 10% preferred using chatbots or AI agents to solve IT issues. Traditional support channels like live chat and email—where human interaction is guaranteed—remain far more popular. “Success isn’t just about the technology; it hinges on the humans who remain involved,” Matchett said. Seth Robinson, VP of industry research at CompTIA, agrees. While automation can handle simple requests, complex issues often require a human touch—not just for expertise, but for the comfort and understanding that AI lacks. “AI delivers solutions based on probability, which means mistakes can happen,” Robinson said. “When people encounter an error from an automated system, it often feels like shouting into the void. With humans, mistakes are understood as part of a back-and-forth process, which people are more willing to accept.” Challenges to AI Adoption The road to fully automated IT support is far from smooth. While AI promises speed and efficiency, it’s not without risks. Gartner predicts that by 2027, half of AI projects in IT service desks will fail due to unforeseen costs, risks, or poor returns on investment. “Frustration with AI doesn’t just end with the problem—it spreads to the entire system,” Robinson said. “When the technology fails, it undermines confidence in a way that human error does not.” A Role in Transition Rather than eliminating the IT support role, AI is transforming it. Routine tasks are increasingly automated, freeing up IT professionals to tackle more complex and strategic issues. And while job postings for IT support specialists have fluctuated, demand remains steady. CompTIA’s latest Tech Jobs Report shows that the number of job postings for IT support roles has remained consistent, with the highest demand occurring as recently as September 2024. “The aggregate picture suggests strong demand for this critical role for the foreseeable future,” Robinson said. The Bottom Line AI is undoubtedly reshaping the IT support landscape, streamlining processes, and boosting efficiency. But human expertise remains indispensable—for now. As workflows evolve and businesses strike a balance between automation and human interaction, the IT support desk is transforming, not disappearing. For employees accustomed to reaching out for help, the shift to AI will take some getting used to. For businesses, it’s a balancing act between embracing innovation and maintaining trust. The future of IT support, it seems, will rely on both man and machine.
Can Robots Learn from Machine Dreams?
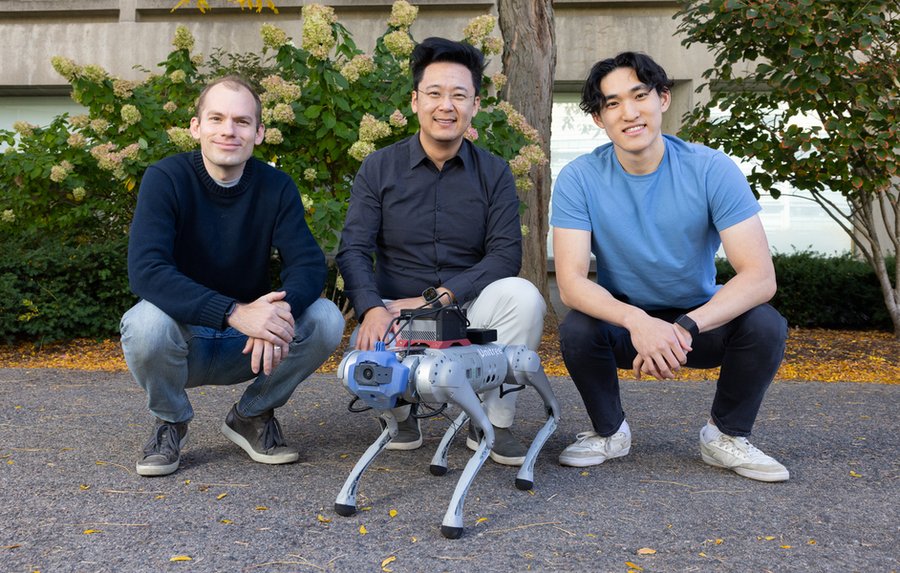
Robotics has always faced a towering challenge: generalization. Building machines that can adapt to unpredictable environments without hand-holding has stymied engineers for decades. Since the 1970s, the field has evolved from rigid programming to deep learning, teaching robots to mimic and learn directly from human actions. Yet, even with this progress, one major bottleneck remains—data. Robots need more than just mountains of data; they need high-quality, edge-case scenarios that push them beyond their comfort zones. Traditionally, this kind of training has required human oversight, with operators meticulously designing scenarios to challenge robots. But as machines grow more sophisticated, this hands-on approach becomes impractical. Simply put, we can’t produce enough training data to keep up. Enter LucidSim, a new system MIT’s Computer Science and Artificial Intelligence Laboratory (CSAI developedL). It leverages generative AI and physics simulators to create hyper-realistic virtual environments where robots can train themselves to master complex tasks—without touching the real world. What Makes LucidSim a Game Changer? At its core, LucidSim addresses one of robotics’ biggest hurdles: the “sim-to-real gap”—the divide between training in a simplified simulation and performing in the messy, unpredictable real world. “Previous approaches often relied on depth sensors or domain randomization to simplify the problem, but these methods missed critical complexities,” explains Ge Yang, a postdoctoral researcher at CSAIL and one of LucidSim’s creators. LucidSim takes a radically different approach by combining physics-based simulations with the power of generative AI. It generates diverse, highly realistic visual environments, thanks to an interplay of three cutting-edge technologies: Dreams in Motion: A Virtual Twist One particularly innovative feature of LucidSim is its “Dreams In Motion” technique. While previous generative AI models could produce static images, LucidSim goes further by generating short, coherent videos. Here’s how it works: The system calculates pixel movements between frames, warping a single generated image into a multi-frame sequence. This approach considers the 3D geometry of the scene and the robot’s shifting perspective, creating a series of “dreams” that robots can use to practice tasks like locomotion, navigation, and manipulation. This method outperforms traditional techniques like domain randomization, which applies random patterns and colors to objects in simulated environments. While domain randomization creates diversity, it lacks the realism that LucidSim delivers. From Burritos to Breakthroughs Interestingly, LucidSim’s origins trace back to a late-night brainstorming session outside Beantown Taqueria in Cambridge, Massachusetts. “We were debating how to teach vision-equipped robots to learn from human feedback but realized we didn’t even have a pure vision-based policy to start with,” recalls Alan Yu, an MIT undergraduate and LucidSim’s co-lead author. “That half-hour on the sidewalk changed everything.” From those humble beginnings, the team developed a framework that not only generates realistic visuals but scales training data creation exponentially. By sourcing diverse text prompts from OpenAI’s ChatGPT, the system generates a variety of environments, each designed to challenge the robot’s abilities. Robots Becoming the Experts To test LucidSim’s capabilities, the team compared it to traditional methods where robots learn by mimicking expert demonstrations. The results were striking: “And the trend is clear,” says Yang. “With more generated data, the performance keeps improving. Eventually, the student outpaces the expert.” Beyond the Lab While LucidSim’s initial focus has been on quadruped locomotion and parkour-like tasks, its potential applications are far broader. One promising avenue is mobile manipulation, where robots handle objects in open environments. Currently, such robots rely on real-world demonstrations, but scaling this approach is labor-intensive and costly. “By moving data collection into virtual environments, we can make this process more scalable and efficient,” says Yang. Stanford University’s Shuran Song, who was not involved in the research, highlights the framework’s broader implications. “LucidSim provides an elegant solution to achieving visual realism in simulations, which could significantly accelerate the deployment of robots in real-world tasks.” Paving the Way for the Future From a sidewalk in Cambridge to the forefront of robotics innovation, LucidSim represents a leap forward in creating adaptable, intelligent machines. Its combination of generative AI and physics simulation could redefine how robots learn and interact with the real world. Supported by a mix of academic and industrial funding—from the National Science Foundation to Amazon—the MIT team presented their groundbreaking work at the recent Conference on Robot Learning (CoRL). LucidSim doesn’t just help robots dream—it helps them learn to navigate our complex, dynamic world without ever stepping into it. Could this be the future of robotics? If the results so far are any indication, the answer is a resounding yes.
GE HealthCare Unveils Revolutionary AI Tool Aimed at Streamlining Cancer Treatment for Doctors
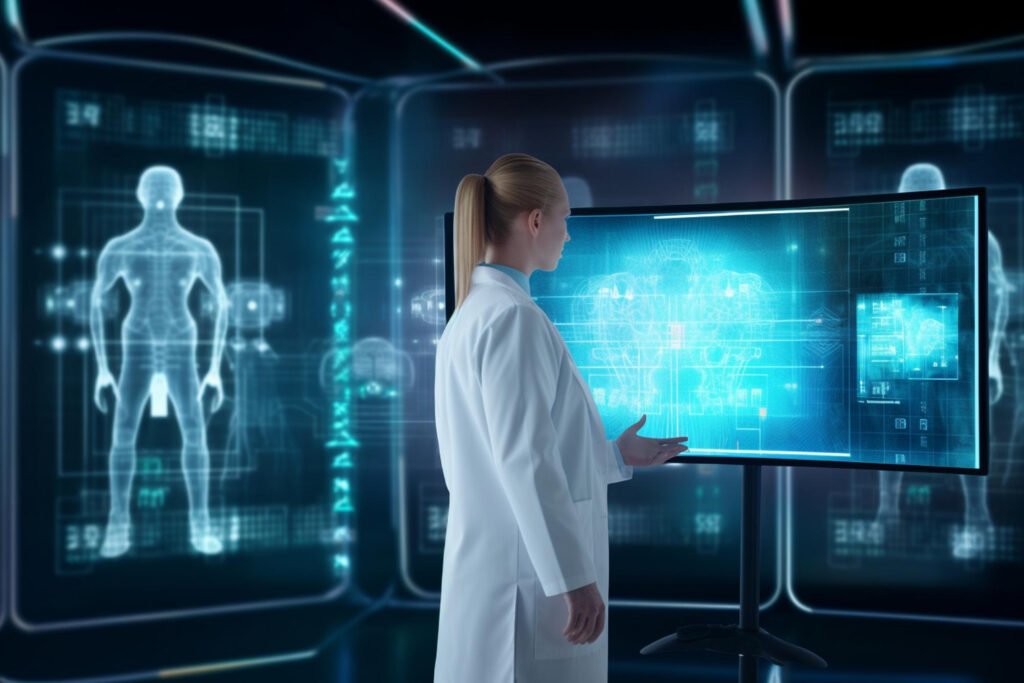
GE Healthcare has unveiled a new AI tool, CareIntellect for Oncology, to save doctors time when diagnosing and treating cancer. This application gives oncologists quick access to critical patient data, allowing them to focus more on patient care than sifting through complex medical records. Doctors have long struggled with analyzing large amounts of healthcare data. A Deloitte report highlights that 97% of hospital data often goes unused, leaving physicians to manually sort through multiple formats like images, lab results, clinical notes, and device readings. CareIntellect for Oncology aims to solve this issue by helping doctors navigate the overload efficiently. “It’s very time-consuming and frustrating for clinicians,” said Dr. Taha Kass-Hout, GE HealthCare’s global chief science and technology officer, in an interview with CNBC. The new tool will summarize clinical reports and flag deviations from treatment plans, like missed lab tests, so that doctors can intervene quickly. AI Solutions Oncology treatments are complicated and can span several years with numerous doctor visits. CareIntellect for Oncology helps physicians track their patients’ journeys, freeing them from administrative tasks. According to Kass-Hout, it also assists in identifying clinical trials for cancer patients, a process that typically takes hours of work. “We’ve removed that burden,” said Chelsea Vane, GE HealthCare’s vice president of digital products. This feature alone could save doctors significant time, allowing them to concentrate on more pressing matters. CareIntellect for Oncology also gives doctors flexibility. They can view original records for deeper insights, ensuring access to detailed patient data when needed. The tool will be available to U.S. customers in 2025, focusing first on prostate and breast cancers. Institutions like Tampa General Hospital are already testing the cloud-based system, which will generate recurring revenue for GE HealthCare. The company also plans to expand CareIntellect’s capabilities with additional AI tools. GE Healthcare is developing five more AI products, including an AI team, to help doctors make quicker, more informed decisions. One tool aims to predict aggressive breast cancer recurrences, while another flags suspicious mammograms for radiologists. These AI-powered innovations aim to ease the burden on clinicians and elevate patient care. Kass-Hout believes these tools can provide the same level of support as a multidisciplinary team, with the added benefit of instant availability. “Our goal is to raise the standard of care and relieve overburdened clinicians,” said Kass-Hout. With CareIntellect for Oncology, GE HealthCare is taking a significant step in using AI to support medical professionals and improve patient outcomes. This technology could shape the future of cancer care, offering hope to doctors and patients.
What Does the Future Hold for Artificial Intelligence?
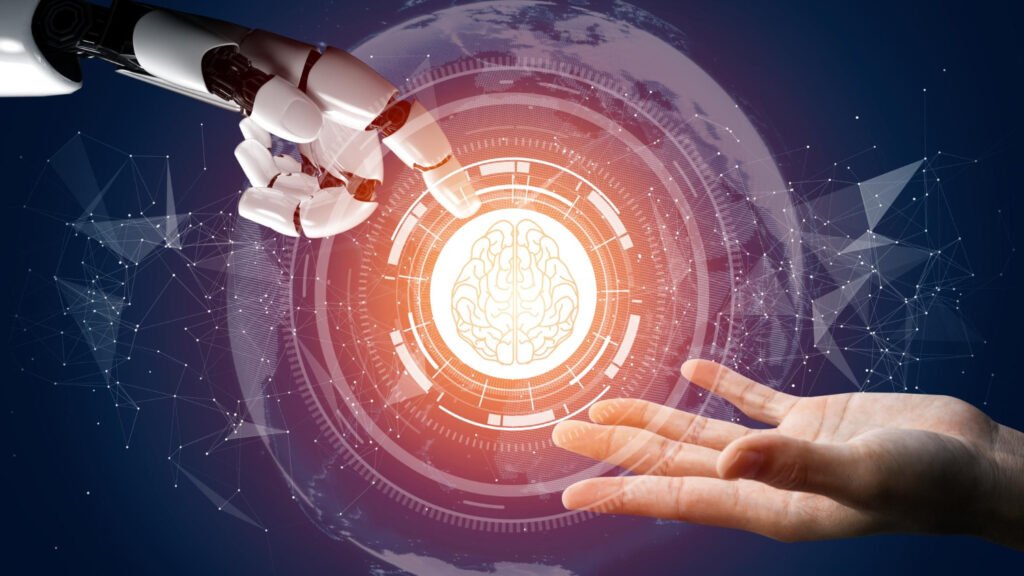
Artificial Intelligence? A few years ago, if someone had told you that by 2024, you could have a conversation with a computer that feels almost completely human, would you have believed them? So, what do you imagine AI will be capable of in the next ten, twenty, or even fifty years? While we can’t predict the future with certainty, it’s safe to assume that many things that seem impossible today will likely become everyday realities. It’s clear now that AI is the defining breakthrough technology of our time. And like other revolutionary technologies before it—fire, steam power, electricity, and computing—AI will continue to evolve and expand its capabilities. One unique aspect of AI is its ability to improve itself. As AI helps create more advanced AI, progress may accelerate in unprecedented ways. So, where could this lead? While these ideas are speculative rather than predictions, pondering the possibilities is intriguing. Let’s embark on a journey into the fascinating, automated, and perhaps not always entirely wonderful world of the future. Future AI Milestones Futurists suggest that several key milestones will mark AI’s evolution. Each will represent a significant leap forward in machine intelligence and its challenges and risks. While the exact timeline is uncertain, this might be the order in which these milestones could arrive: Artificial General Intelligence (AGI):AGI refers to machines that can apply their knowledge and learning across various tasks. Unlike today’s “narrow” or “specialized” AI, designed for specific tasks, true generalized AI will think, act, and solve problems more like humans, especially in areas requiring creativity and complex problem-solving. Quantum AI:This milestone involves the convergence of quantum physics, which deals with sub-atomic particles and AI. Quantum computing, still in its infancy, can perform some computations up to 100 million times faster than classical computing. In the future, quantum AI could supercharge algorithms, enabling them to process massive datasets and solve complex problems like optimization and cryptography at unprecedented speeds. The Singularity:This is the hypothetical point where AI surpasses human intelligence and begins to improve itself autonomously and exponentially. The outcomes are unpredictable, as AI may develop ideas and make decisions that differ significantly from human expectations. Consciousness?The ultimate milestone could be machines so sophisticated that they possess self-awareness and experience reality like humans. This is a deeply philosophical question; some believe it may never truly happen—or we might not recognize it even if it does. Insect brains, for example, are more complex than the most advanced AI, yet we still debate whether they are conscious. If machines achieve consciousness, it will open a whole new set of ethical dilemmas for society. Glimpses of the Future Now that we have a rough roadmap, let’s fast-forward to see what these developments might mean for life in the mid- to distant future. Ten Years From Now: In 2034, Tom, a 45-year-old with a family history of heart disease, uses an AI health assistant implanted under his skin to monitor his vital signs and nutrient levels. This AI provides early warnings and personalized health recommendations based on his genetic makeup. By this time, healthcare has become more preventative, helping everyone live healthier lives and significantly reducing the societal cost of sickness. Twenty Years From Now: In 2044, Tom’s daughter Maria, a recent graduate, works as a climate engineer. Her primary task is to mitigate the impact of climate change worldwide. She relies heavily on AI technology to monitor, predict, and manage environmental conditions. Her highly strategic work involves AI-driven solutions and collaboration with other professionals to foresee and address future threats. Thanks to advances in AI and biotechnology, Maria doesn’t have to worry about her family’s history of heart disease, as the genetic fault was corrected before she was born. Fifty Years From Now: In 2074, Carlos lives in a sprawling megacity where everything he does is monitored and analyzed by machines to ensure compliance with strict laws and environmental regulations. Data from surveillance cameras, online activity, and personal tracking devices monitor energy use, waste production, and carbon footprint. AI algorithms analyze this data, rewarding compliant citizens with credits for rationed goods and travel while restricting luxuries and freedoms for those who fail to comply. It’s a dystopian reality. Seventy Years From Now: Welcome to the post-scarcity economy—a time of abundance. By 2094, self-propagating AI will solve society’s biggest challenges. Aiko, now an adult, will never experience poverty or lack access to food, shelter, and healthcare. Automated manufacturing and 3D printing have dramatically reduced the cost of producing essential items, and AI-managed agriculture ensures that no one goes hungry. Most importantly, Aiko will never have to work—robots manage the industry and economy, allowing humans to indulge in creative and leisurely pursuits. One Hundred Years From Now: Future AI, Artificial Intelligence Nova is among the first residents of a permanent Martian colony in 2124. Intelligent, lifelike robots are part of everyday life, performing physical labor and serving as companions and personal assistants. Thanks to her neural interface, Nova has direct access to powerful AI that enhances her cognitive abilities, making her far smarter than her ancestors a century ago. She will also live much longer, thanks to AI-developed biotechnology that significantly extends her lifespan and eliminates most of the risks of illness. Humanity is just beginning to explore the stars, knowing they aren’t the only intelligent entities in the universe. But Seriously While some of these scenarios might sound more like science fiction than reality, remember that many of today’s AI tools would have seemed as unbelievable only a few years ago. As we progress, one thing is sure: the rapid pace of technology-driven change will continue to blur the lines between fiction and reality. Ideas that seem far-fetched today could become commonplace for our children or grandchildren. After all, excellent science fiction entertains us and helps us consider the ethical and societal challenges that lie ahead. Self-aware robots, AI-powered immortality, an end to sickness, inequality, and poverty, or a solution to the climate crisis—thanks to AI, all of these are possibilities.
What It Truly Takes to Train an Entire Workforce on Generative AI
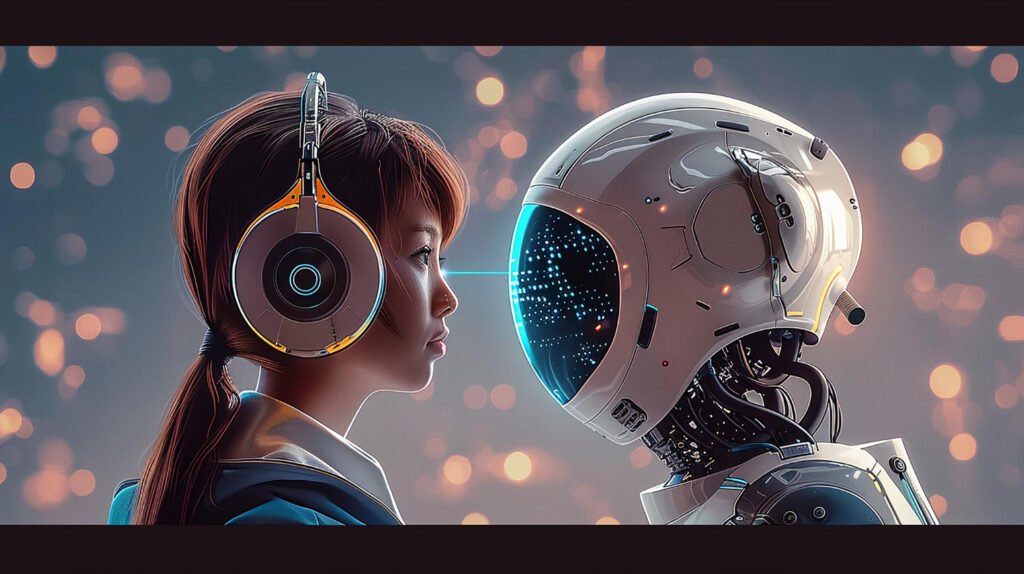
Generative AI: In the rapidly evolving landscape of upskilling, companies with vast workforces face significant challenges, particularly when training employees on generative artificial intelligence (AI). This task is especially daunting given the novelty of the technology. However, many organizations are embracing the challenge, recognizing the importance of AI for both operational efficiency and long-term employee success. According to Microsoft’s 2024 Work Trend Index, 66% of leaders would hesitate to hire new employees without AI skills, underscoring the urgency of this training. As organizations embark on this widespread AI training, they are learning valuable lessons along the way. Take Synechron, a global IT services and consulting company, as an example. With a workforce of approximately 13,500 employees, most are now AI-enabled, thanks to a well-planned training initiative. Given the regulated environments in which many clients operate, Synechron developed nine secure internal solutions, including a ChatGPT-like application called Nexus Chat. Among employees not working at restricted client sites, 84% actively use Nexus Chat. AI, Generative AI Synechron’s Chief Technology Officer David Sewell explains that access to these tools was the first step in their training process. The company began with an online course focused on beginner-level prompt engineering, teaching employees how to interact with AI effectively. Additionally, Synechron produced videos demonstrating potential use cases for non-technical roles, such as those in human resources or legal departments, and included questionnaires to accelerate proficiency. During a trial period, a select group of technologists and general employees were granted early access to these tools, including Unifai, an AI-powered human resources bot designed to handle sensitive HR policies and company data. Today, 74% of employees are using Unifai. On the technical side, Sewell reports a 39% increase in productivity within the software development lifecycle. Although the impact on non-technical roles is harder to quantify, Synechron’s Chief Marketing Officer, Antonia Maneta, shares, “After just a few months, I can’t imagine running my business without AI. It’s transformed our productivity, allowing us to focus on the most critical tasks.” Amala Duggirala, Chief Information Officer at financial services company USAA, is developing an AI training program for 37,000 employees. Her strategy centers on three key steps. First, governance and risk management are prioritized. Next, senior leaders undergo training on solutions that have passed governance analysis, with sessions led by industry experts. Finally, different teams receive tailored educational courses based on their roles, whether they are involved in creating technology, safeguarding the organization from risks, or simply using AI tools. Tech, Generative AI Hackathons are another effective tool for hands-on AI experience. USAA’s recent hackathon saw record participation from technical and non-technical teams, reflecting widespread enthusiasm. The event generated 55 new use case ideas, which are now being tested in a controlled environment. “The level of interest across the organization was astounding,” Duggirala notes. Similarly, Synechron hosted a hackathon but received feedback that some participants didn’t feel fully prepared. In response, the company developed additional training materials, giving employees more time to familiarize themselves with the technology before expecting measurable results. Workforce, Generative AI Different companies, however, approach AI training in ways that best suit their specific needs. Terry O’Daniel, Head of Security at digital analytics platform Amplitude, emphasizes the importance of clear guidelines and practical solutions over comprehensive training. With experience at companies like Instacart, Netflix, and Salesforce, O’Daniel focuses on data privacy, security, intellectual property, and output verification, ensuring employees are informed about using AI responsibly. At Amplitude, which has over 700 employees, O’Daniel’s team encourages employees to seek approval before implementing new AI solutions, using the company’s corporate subscription to the OpenAI API feed rather than public platforms that could compromise data security. For larger companies like USAA and Synechron, more structured approaches are necessary. Synechron’s Head of AI, Ryan Cox, travels to global offices, identifying enthusiastic employees who can advocate for AI training within their local teams. This structured evangelization is key to ensuring responsible AI usage across the organization. Ultimately, while the approach to AI training varies, the common thread is the necessity of responsible AI usage. As Duggirala of USAA aptly says, “We will fall behind if we don’t embrace AI, but we will fall even further behind if we don’t approach it responsibly.”
A new wave of African talent is leveraging cutting-edge AI to tackle scientific challenges.
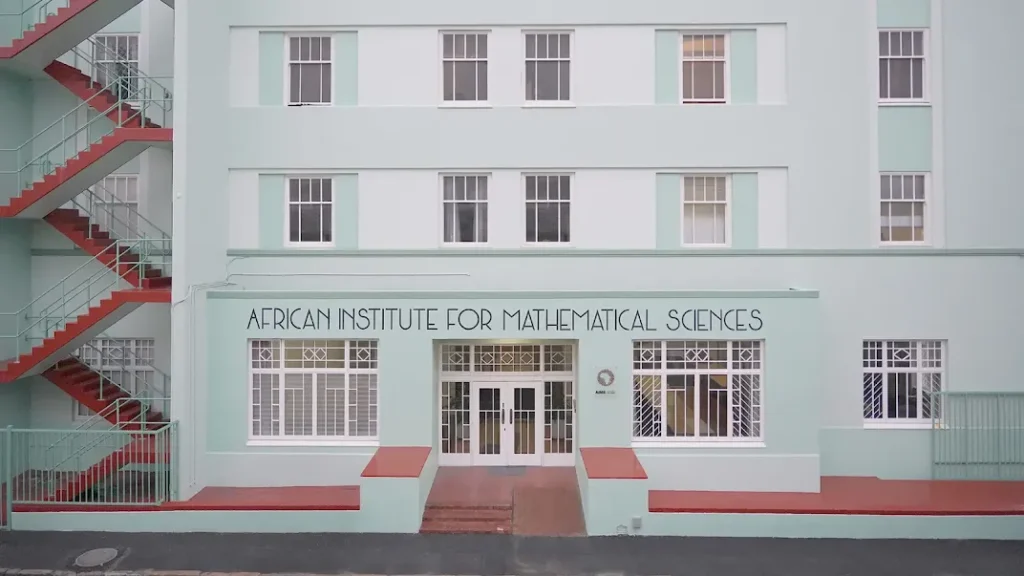
Students of a new pan-African Master’s program aspire to apply AI in food security, healthcare, and exploring the cosmos. At Google DeepMind, our mission is to nurture the next generation of AI leaders and foster a more diverse and inclusive global AI community through education. Our commitment includes enhancing access to AI and science. AI, Africa In partnership with the African Institute for Mathematical Sciences (AIMS), Africa’s pioneering network of centers of excellence in mathematical sciences, we launched an AI for Science Master’s program with a $4.5M grant from Google DeepMind. This funding enables AIMS to provide full scholarships, equipment, and computing resources to talented local students, facilitating advanced mathematics, AI, and machine learning studies at AIMS South Africa. With mentoring and support from Google DeepMind’s researchers and engineers, students are poised to accelerate scientific discovery. This summer, the first cohort of students graduated at a ceremony held at the AIMS campus in Cape Town, South Africa. As emerging AI leaders in Africa, Béria Chingnabé Kalpélbé, Olivier Mahumawon Adjagba, and Diffo Mboudjiho Annette Dariose shared their experiences in pioneering AI research and their aspirations for their work. Béria Chingnabé Kalpélbé: Innovating for Food Security and Sustainability Béria, from Chad, is dedicated to applying AI to sustainability challenges. “My goal is to develop solutions for sustainable agricultural development that benefit both people and the planet by integrating principles of renewable energy, precision farming, and ecological preservation,” he says. Béria believes AI has tremendous potential to enhance the resilience of Africa’s natural environments. “By implementing AI-powered monitoring and decision-support systems, we can safeguard Africa’s precious green areas and biodiversity for future generations.” Olivier Mahumawon Adjagba: Advancing Virus Transmission Research Amid Climate Change cutting-edge AI Olivier, from Benin, has a passion for applying mathematics to complex problems, leading him to AIMS South Africa. “Throughout my academic journey, I’ve been fascinated by the power of mathematics in addressing real-world challenges through AI,” he shares. With a strong foundation in mathematical sciences, Olivier aims to drive progress in healthcare, climate science, and technology. Olivier now focuses on using AI to understand the spread of dengue fever. “Using advanced AI techniques, I hope to create more accurate prediction models to inform public health strategies and interventions, ultimately contributing to the control and prevention of this viral disease.” On his scholarship, Olivier notes, “Without it, pursuing advanced studies at such a prestigious institution would have been financially unattainable. This support enabled me to immerse myself in AIMS’ rigorous academic environment, collaborate with professors and peers, and contribute meaningfully to research projects.” Diffo Mboudjiho Annette Dariose: Exploring the Universe with AI Diffo, from Cameroon, is captivated by the mysteries beyond Earth, which drew her to the Square Kilometre Array (SKA), the world’s largest and most sensitive radio telescope. “Understanding the 21cm line offers insights into the early universe, the formation of the first stars and galaxies, and the structure of the cosmos,” she explains. By applying Markov chain Monte Carlo (MCMC) techniques, Diffo aims to improve the accuracy and efficiency of extracting these faint signals from SKA data, potentially leading to more precise cosmological models and a deeper understanding of the universe’s future evolution. For those considering similar studies, Diffo advises: “Stay curious, be persistent, and embrace interdisciplinary learning. Engaging in hands-on projects, collaborating with peers, and seeking mentorship from AI experts can greatly enhance your learning experience and career prospects.” Supporting AI Education in Africa AI, Africa Our efforts build on existing commitments in the region, including support for the Deep Learning Indaba through volunteering and funding since 2017 and the launch of our Experience AI education program across Africa, which has engaged over 30,000 young people. Additionally, educational funding has enabled three more African universities to offer over 40 postgraduate scholarships since 2020. Increasing representation in AI research brings diverse values, perspectives, and concerns into the conversation about this transformative technology. Our support for AIMS aims to build a more global and inclusive AI ecosystem, helping students make scientific discoveries that benefit their local communities and the world.