AI-Powered Predictive Maintenance in Manufacturing: Maximize Equipment Reliability
Predictive maintenance (PdM) is a data-driven approach that helps manufacturers anticipate equipment failures before they happen. This specialized strategy uses advanced data analysis and condition monitoring to maintain equipment only when necessary rather than on a fixed schedule. This shift optimizes machine uptime, lowers costs, and extends asset lifespan.
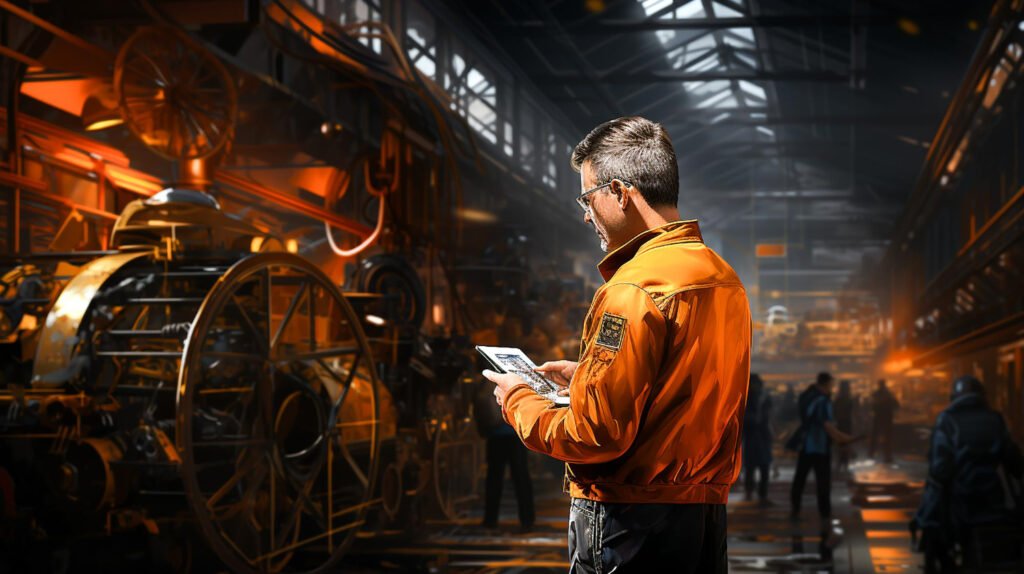
What Makes Predictive Maintenance Specialized?
Predictive maintenance stands out because it integrates the following:
- Condition Monitoring Techniques – Methods like vibration analysis, thermography, oil analysis, and ultrasound detect early signs of wear or failure.
- Advanced Data Analytics – Machine learning models analyze sensor data, detecting patterns and anomalies missed by traditional methods.
- Real-Time Monitoring – Sensors continuously track temperature, vibration, and electrical use, alerting technicians to changes.
- Prognostics and Health Management (PHM): PHM helps predict a machine’s remaining helpful life (RUL) and suggests when maintenance should occur.
Techniques in Predictive Maintenance
Predictive maintenance uses several core techniques:
- Vibration Analysis – Detects issues like misalignment or imbalance in rotating equipment.
- Infrared Thermography – Shows heat patterns, revealing electrical or lubrication issues.
- Oil Analysis – Identifies contaminants and wear particles in lubricant samples.
- Ultrasonic Testing – Detects leaks or stress not heard by the human ear.
- Electrical Monitoring – Analyzes power use to find faults in motors and pumps.
Technologies Powering Predictive Maintenance
Predictive maintenance relies on the following:
- Industrial IoT (IIoT) – Connects equipment sensors to networks for seamless data sharing.
- Machine Learning and AI – Analyze historical and real-time data to predict failures.
- Digital Twins – Simulates equipment behavior to anticipate potential issues.
- Big Data Analytics – Processes large datasets for actionable insights.
Benefits of a Predictive Maintenance Approach
This specialized approach delivers:
- Targeted Maintenance – Reduces downtime by performing maintenance only as needed.
- Extended Equipment Life – Prevents minor issues from escalating.
- Optimized Costs – Cuts costs by reducing emergency repairs and spare parts inventory.
- Improved Quality Control – Ensures machinery operates within specified tolerances.
- Enhanced Safety – Addresses issues before they become hazardous.
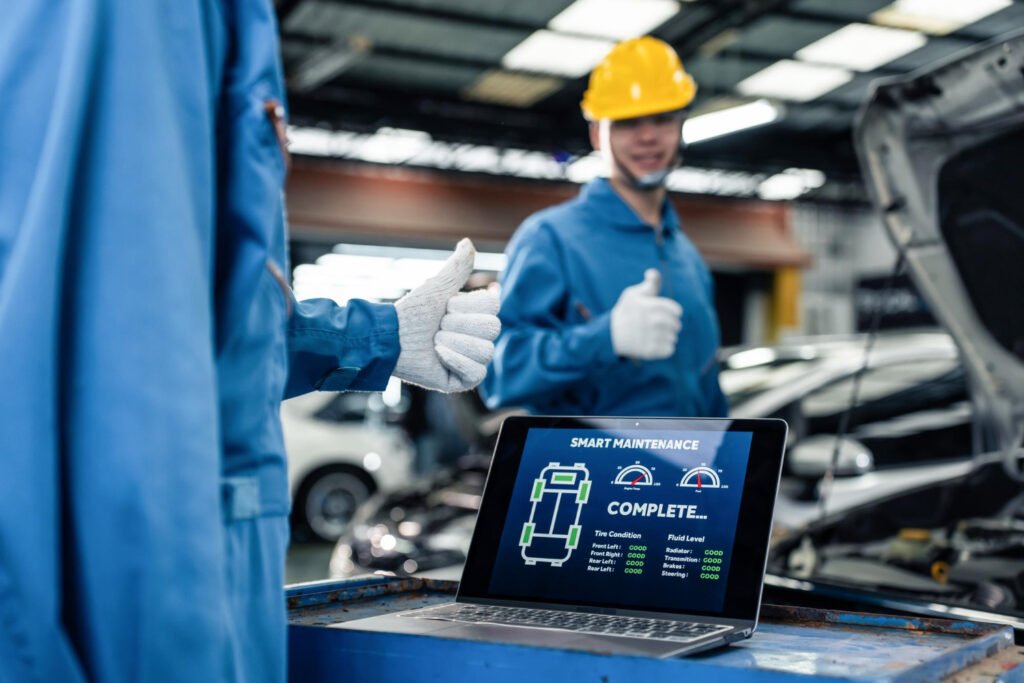
Challenges in Implementing Predictive Maintenance
Predictive maintenance also has challenges:
- Setup Costs – Initial investments in sensors and software are high.
- Data Quality – Reliable data is crucial for accurate predictions.
- Legacy Systems – This may require upgrades to support predictive maintenance.
- Skills Gap – Requires expertise in data science and machine learning.
Best Practices for Implementation
To succeed with predictive maintenance:
- Start with Critical Equipment – Focus on key assets that impact production.
- Use a Step-by-Step Approach – Pilot programs allow for initial testing.
- Ensure Data Integration – Consolidate sensor data on a centralized platform.
- Train Staff – Invest in training to maximize program benefits.
- Continuously Improve – Update models regularly to refine accuracy.
The Future of Predictive Maintenance in Manufacturing
With advancements in Industry 4.0, AI, and machine learning, predictive maintenance will offer even more specialized insights into equipment health. This proactive approach is not just a trend but essential for modern manufacturing. Manufacturers can unlock efficiency, sustainability, and profitability at new levels by adopting predictive maintenance.